AI Showdown: Open-Source Models Cry Foul Over Leaderboard Bias
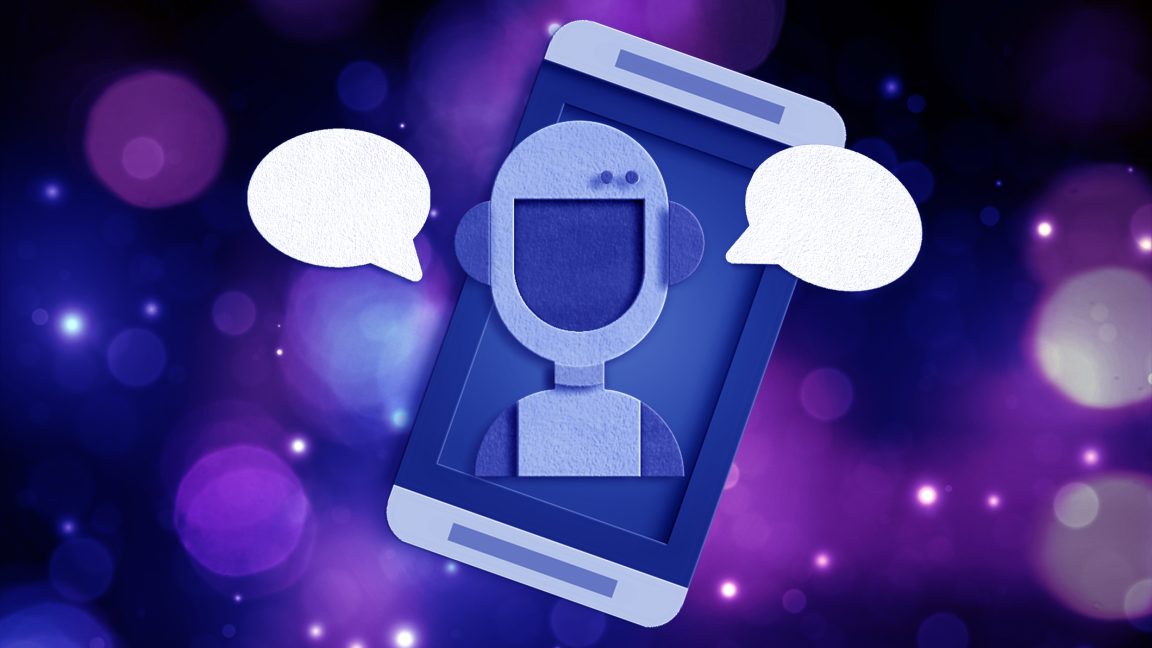
The AI Vibe Test: Unmasking Hidden Biases in Algorithmic Assessments
In the rapidly evolving world of artificial intelligence, the seemingly innocuous "vibe test" has become a trendy tool for evaluating personalities and potential. However, beneath its sleek digital surface lies a complex web of potential discrimination and unintended bias that demands closer scrutiny.
Recent investigations have revealed that these AI-powered assessments may not be the neutral arbiters of judgment they claim to be. Instead, they often reflect deeply ingrained societal prejudices, inadvertently perpetuating systemic inequalities through their algorithmic frameworks.
The core issue stems from the training data used to develop these AI systems. When machine learning models are fed historical data that contains existing social biases, they don't just replicate information—they amplify and normalize these biases. This means that marginalized groups can face disproportionate challenges in passing these seemingly objective tests.
Experts warn that what appears to be a simple personality assessment can have far-reaching consequences. From job recruitment to social interactions, these AI vibe tests can potentially limit opportunities for individuals based on factors beyond their control.
As technology continues to integrate deeper into our daily lives, it becomes crucial to demand transparency, accountability, and continuous refinement of these algorithmic tools. The goal should be creating AI systems that truly represent fairness and equal opportunity for all.
The conversation around AI bias is not about condemning technology, but about ensuring that our technological advances reflect the best of human potential—inclusive, empathetic, and just.