Numbers That Predict Millions: How AI Is Reshaping Wall Street's Crystal Ball
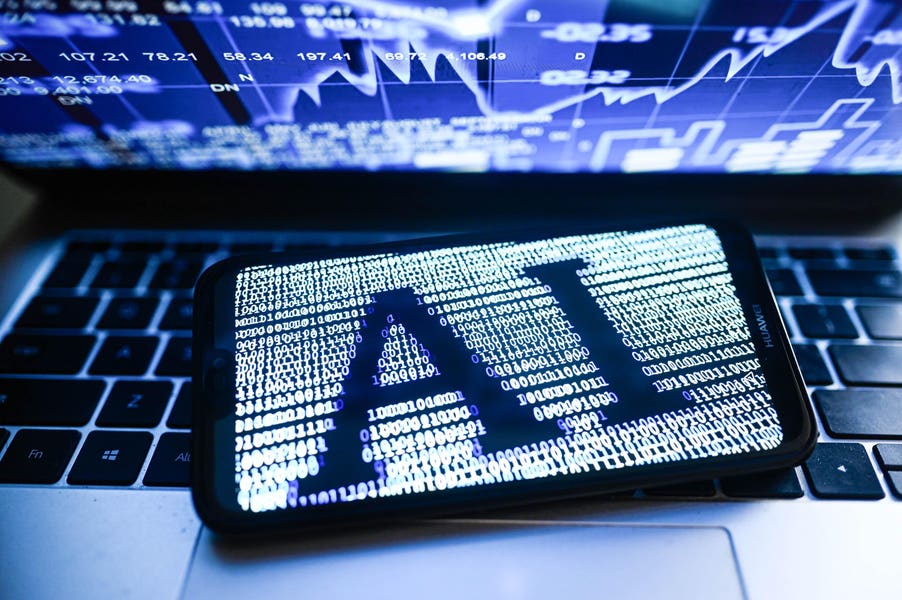
In the fascinating world of artificial intelligence, statistical methods serve as the foundational building blocks for developing sophisticated Large Language Models (LLMs). Descriptive and inferential statistics are not just mathematical tools, but powerful techniques that breathe life into machine learning algorithms.
Descriptive statistics act as the initial lens through which researchers understand complex data landscapes. By summarizing and organizing massive datasets, these methods help data scientists extract meaningful patterns and insights. Mean, median, standard deviation, and variance become critical metrics that reveal the underlying structure of linguistic data, enabling more precise model training.
Inferential statistics take this understanding a step further, allowing researchers to make robust predictions and draw meaningful conclusions from sample data. Through techniques like hypothesis testing and confidence intervals, data scientists can generalize findings and validate the statistical significance of their model's performance.
In the context of LLMs, these statistical methods play multiple crucial roles:
1. Data Preprocessing: Identifying and handling outliers
2. Model Validation: Assessing model reliability and generalizability
3. Performance Measurement: Quantifying model accuracy and precision
4. Error Analysis: Understanding and minimizing statistical variations
By leveraging these fundamental statistical approaches, researchers can develop more intelligent, nuanced, and reliable language models that push the boundaries of artificial intelligence and natural language processing.